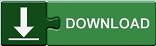
In the case of crops classification for satellite images the number of features is high, which is a limitation for the direct use of the GMMF. However, the computation of the likelihood becomes a very hard problem when the number of features increases. In the case of 1D or 3D feature spaces the likelihood can be computed based on the corresponding normalized histograms. One of the main difficulties for GMMF, as for all methods based on the combination of Bayesian estimation and MRF for image segmentation, is the likelihood computation. Gauss Markov Measure Field (GMMF) is one of the models that combines Bayesian estimation with Markov Random Field and it is used in many classification tasks. This assumption is specified by considering a Markovian priori distribution. With this approach, a label field is computed assuming that a dependence exists between all probability distributions of the pixels belonging to the same neighborhood. A very effective approach for including the features of the pixel neighborhood is the Bayesian estimation together with the Markov Random Field (MRF).
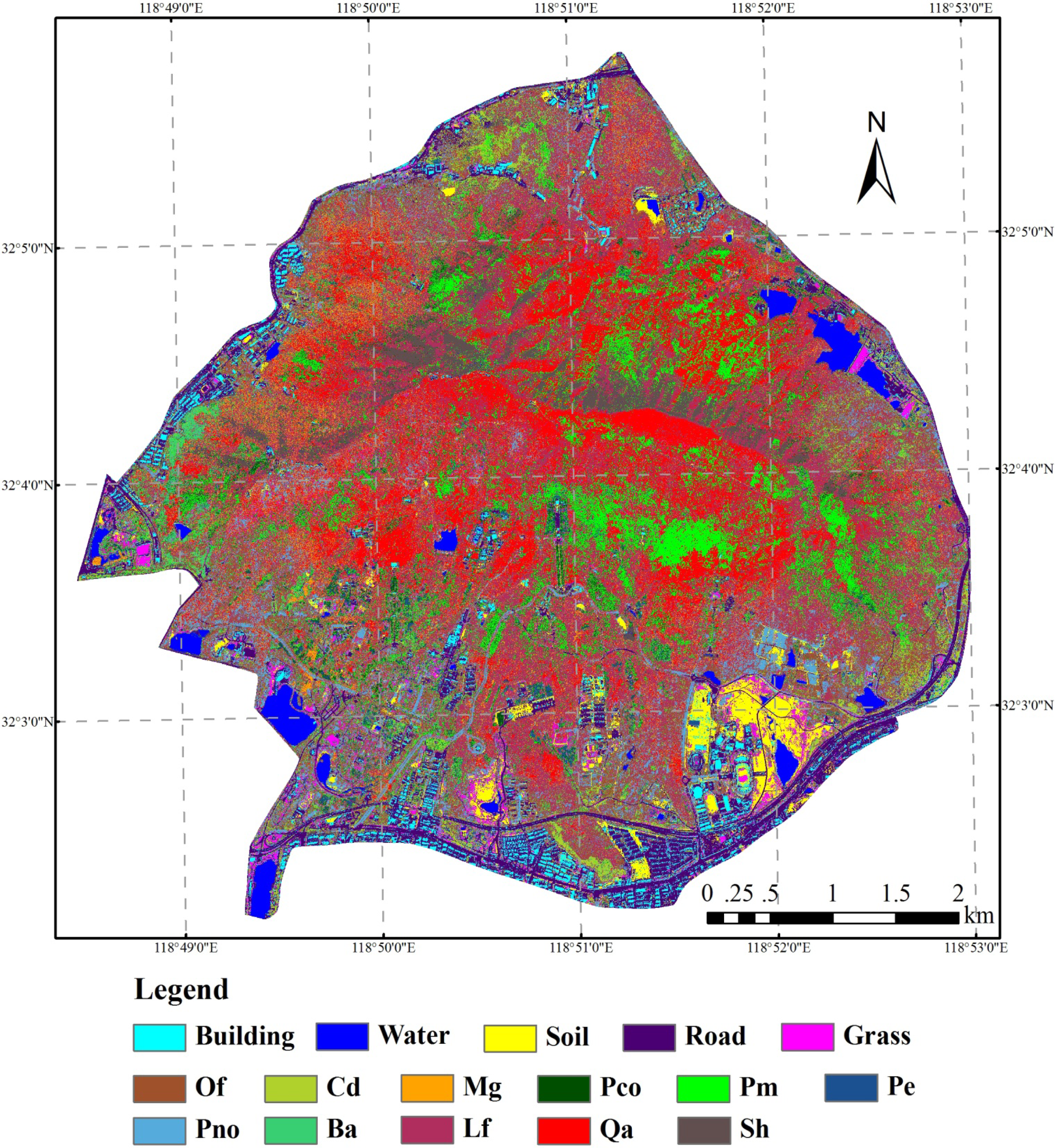
Clustering algorithms such as k-means, fuzzy c-means and the Iterative Self-Organizing Data Analysis Technique (ISODATA) algorithm have been used successfully for segmentation problems, however these methods per se, do not consider the contextual information for a pixel, what is necessary to obtain a good segmentation. The segmentation process allows us to divide the image into significant parts according a certain criterion. The proposed algorithm was applied to a real image and obtained excellent results in comparison to different classification algorithms used in crop classification. Different feature spaces are analyzed, among them are 6 spectral bands from LANDSAT 5 TM, 3 principal components from PCA on 6 spectral bands and 3 principal components from PCA applied on 10 vegetation indices. The computed likelihoods are the main input of the proposed algorithm and are combined in the proposed model using a contrast criteria. Based on previous histograms, we can compute the likelihood of each site of the image to belong to a class. For this purpose, the method requires prior information for building a 3D histogram for each considered feature space. The proposed method allows us to combine several feature spaces.
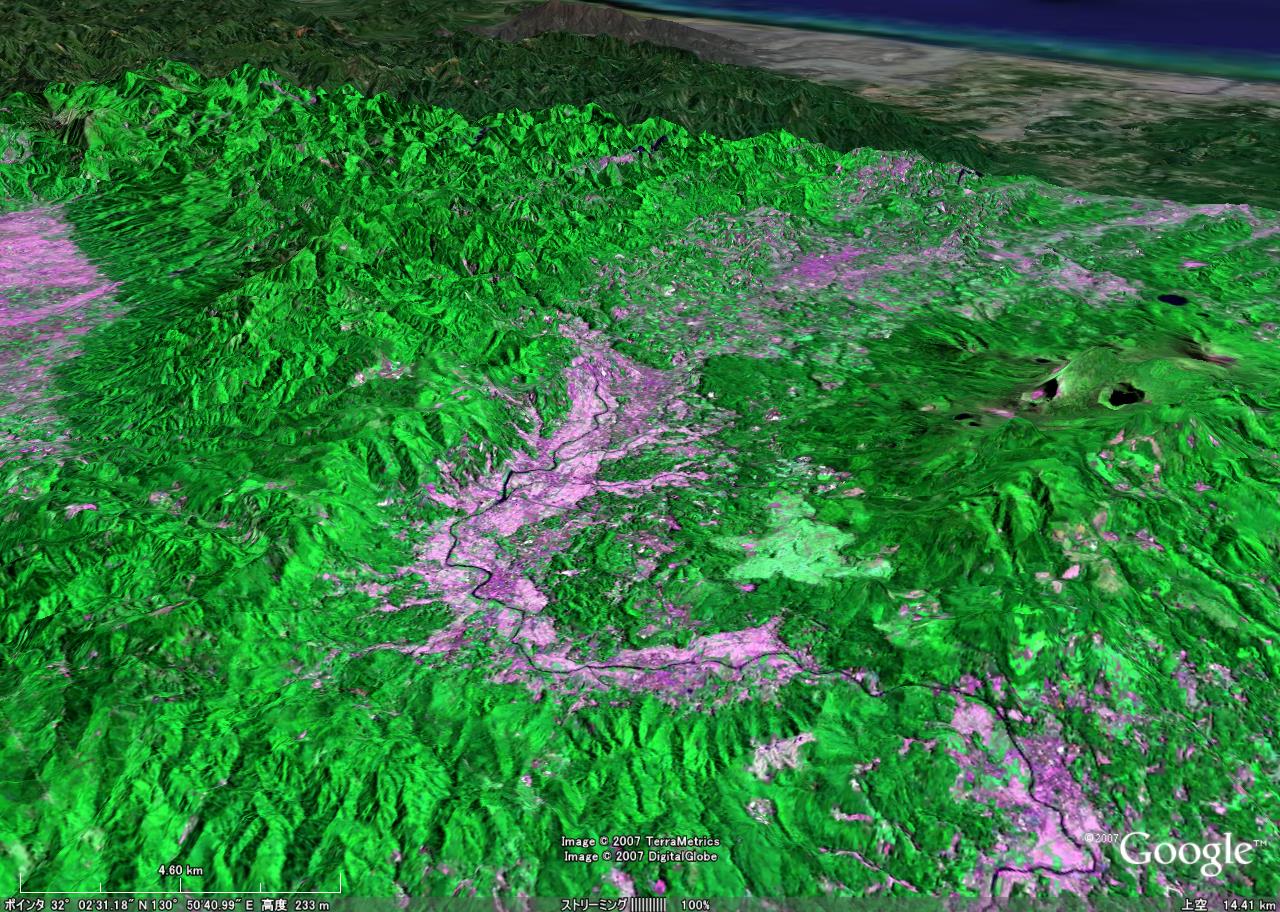
In particular, we apply the general model to classify types of crops in satellite images. In this work, we propose a generalization of Gaussian Markov Measure Field Models and provide a probabilistic segmentation scheme, which fuses multiple information sources for image segmentation. However, when the number of information sources grows, as in the case of satellite images, the histogram construction becomes more difficult due to the high dimensionality of the feature space. Typically, in these methods, the likelihood is computed from 1D or 3D histograms. Classification methods based on Gaussian Markov Measure Field Models and other probabilistic approaches have to face the problem of construction of the likelihood.
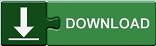